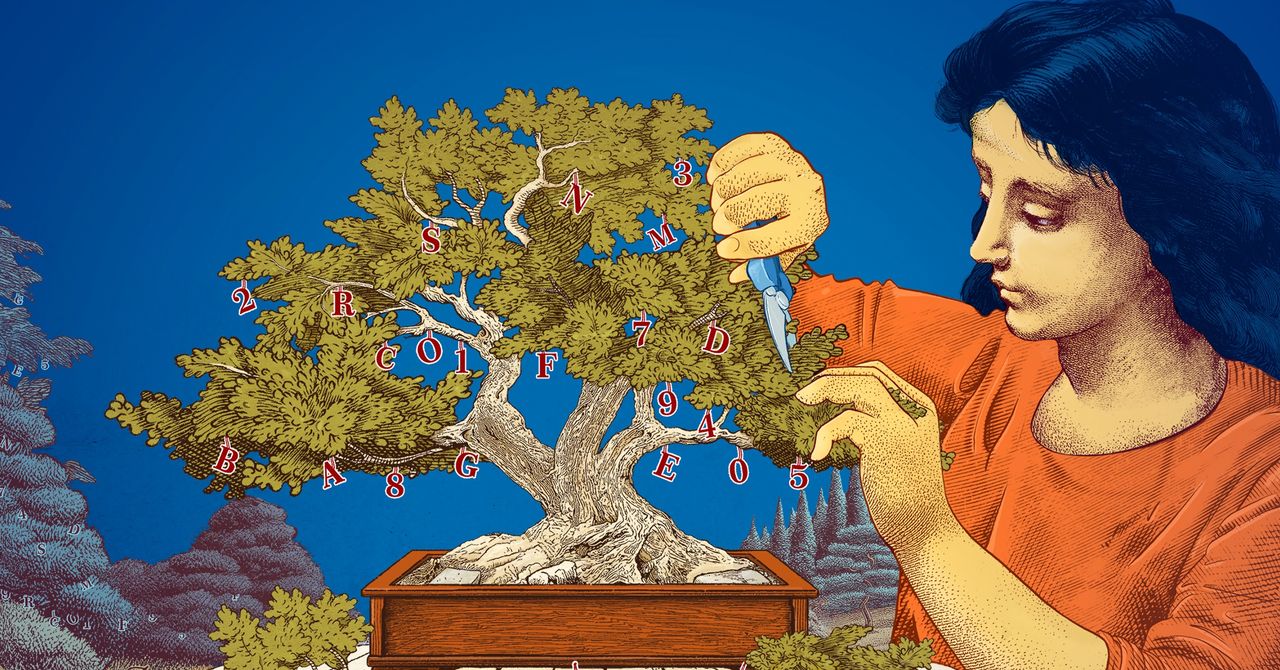
Researchers claim that small language models are in vogue.
Larger models are capable of performing a greater diversity of tasks; however, the compact size of smaller models makes them very attractive tools.
Large language models are effective due to their magnitude. The latest versions developed by OpenAI, Meta, and DeepSeek employ hundreds of billions of "parameters," which are the adjustments that regulate connections between data and which are modified during the training process. As the number of parameters increases, models improve their ability to identify patterns and relationships, making them more powerful and accurate.
However, this power comes at a high cost. To train its Gemini 1.0 Ultra model, Google invested approximately $191 million. Large language models (LLMs) also require substantial computational power every time they generate responses, earning them a reputation as significant energy consumers. According to the Electric Power Research Institute, a single query to ChatGPT consumes around ten times more energy than performing a Google search.
In response to this situation, some researchers have begun seeking smaller alternatives. Recently, IBM, Google, Microsoft, and OpenAI have launched small language models (SLMs), which use a few billion parameters, a fraction of what their larger counterparts utilize. Although these models are not suitable as general-purpose tools, they excel at specific, defined tasks such as summarizing conversations, answering patient questions in health chatbots, and gathering information on smart devices. Zico Kolter, a computer scientist at Carnegie Mellon University, notes that "for many tasks, an 8-billion parameter model is quite effective." Furthermore, these models can run on laptops or mobile phones rather than requiring a large data center (there is no clear consensus on the exact definition of "small," but new models usually cap at around 10 billion parameters).
To optimize the training process of these reduced models, researchers employ various strategies. Large models often draw raw training data from the internet, which can be disorganized and challenging to process. However, these large models can generate a high-quality dataset that serves to train a small model. This approach, known as knowledge distillation, allows the larger model to effectively transfer its learning, akin to a teacher instructing a student. Kolter points out that "the reason why SLMs are so effective despite having few parameters and little data is that they use high-quality data rather than cluttered data."
Researchers have also explored methods for creating small models from large ones, such as the pruning method, which involves removing unnecessary or inefficient components from a neural network. This technique was inspired by the human brain, which becomes more efficient by trimming connections between synapses as a person ages. Current pruning techniques trace back to a 1989 paper by computer scientist Yann LeCun, who argued that up to 90% of parameters in a trained neural network could be eliminated without losing efficiency, describing this approach as "optimal brain damage." Pruning helps researchers fine-tune a small language model for specific tasks or environments.
For scientists interested in understanding how language models work, SLMs represent a cost-effective option for testing innovative ideas. Additionally, having fewer parameters than large models can make their reasoning more transparent. Leshem Choshen, a researcher at the MIT-IBM Watson AI Lab, states that "if you want to create a new model, you need to experiment." Small models allow researchers to test different approaches with lower risks.
While large and costly models will remain relevant for applications like generalized chatbots, image generators, and drug discovery, for many users, a small, focused model can be just as effective, in addition to being easier to train and build. According to Choshen, "these efficient models can save money, time, and computational resources."