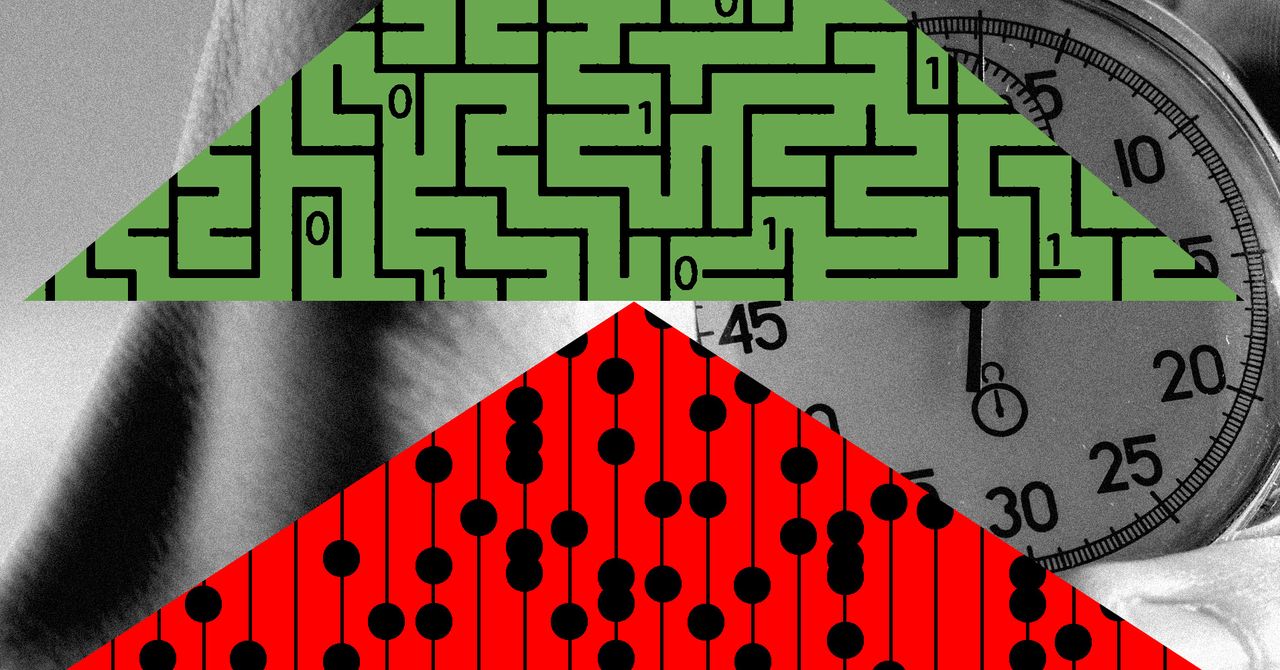
This tool examines cutting-edge AI models for intelligence failures.
A new platform from the data training company Scale AI will allow artificial intelligence developers to identify the weak areas of their models.
Executives of artificial intelligence companies often claim that AGI is just around the corner, but recent models still require additional guidance to reach their full potential. Scale AI, a key player in developing advanced AI models, has created a platform that can automatically evaluate a model through thousands of tests and tasks, identify weaknesses, and highlight additional training data that could enhance its capabilities. Scale will, of course, provide the necessary data for this process.
Scale has gained recognition for offering human labor for the training and evaluation of advanced artificial intelligence models. Large language models (LLMs) are trained using vast volumes of text gathered from books, websites, and other sources. To turn these models into useful, coherent, and polite chatbots, additional training is required from humans who provide feedback on the model's output.
The company provides specialized workers to identify issues and limitations in the models. The new tool called Scale Evaluation automates part of this work using proprietary machine learning algorithms from Scale. According to Daniel Berrios, the product lead for Scale Evaluation, “within large labs, there exist disorganized ways to track some weaknesses in the models.” The new tool allows model creators to analyze results and understand where their models are not performing well, helping them direct data campaigns toward improvement.
Berrios mentions that several companies with cutting-edge AI models are already using this tool, primarily to enhance the reasoning capabilities of their best models. Reasoning in artificial intelligence involves a model breaking down a problem into parts to solve it more effectively, which largely depends on user feedback regarding whether the model has solved a problem correctly.
In a specific case, Berrios notes that Scale Evaluation found that a model's reasoning skills declined when given instructions in languages other than English. "While the reasoning capabilities were generally quite good and performed well on the tests, they tend to degrade significantly when the instructions are not in English," he explains. The tool allowed the company to collect additional training data to address this deficiency.
Jonathan Frankle, chief AI scientist at Databricks, a company that develops large AI models, believes that testing one baseline model against another is useful in principle. “Anyone who contributes to the evaluation is helping us build better AI,” states Frankle.
In recent months, Scale has contributed to the development of several new standards designed to enhance the intelligence of AI models, as well as to analyze more carefully how they might behave inappropriately. These include EnigmaEval, MultiChallenge, MASK, and Humanity's Last Exam. However, the company also notes that measuring improvements in AI models is becoming more complicated as they become more adept at passing existing tests. The new tool provides a more comprehensive view by combining various standards and can be used to develop customized tests of a model's capabilities, such as evaluating its reasoning in different languages.
Additionally, the tool could contribute to efforts to standardize testing of AI models regarding inappropriate behaviors. Some researchers argue that the lack of standardization means that some vulnerabilities in the models remain undisclosed. In February, the U.S. National Institute of Standards and Technology announced that Scale would help develop methodologies for testing models and ensuring that they are safe and trustworthy.